출처: https://cceyda.github.io/blog/torchserve/streamlit/dashboard/2020/10/15/torchserve.html
토치서브 설치, 대시보드 설치, HTTP 요청
Torchserve
Torchserve 예제와 대시보드 사용
torchserve streamlit dashboard
이전 내용 참고: post (transformer model with torchserve to have your own self-hosted token-classification inference widget).
이어서 토치서브를 더 알아보고 torchserve management dashboard도 살펴보겠다.
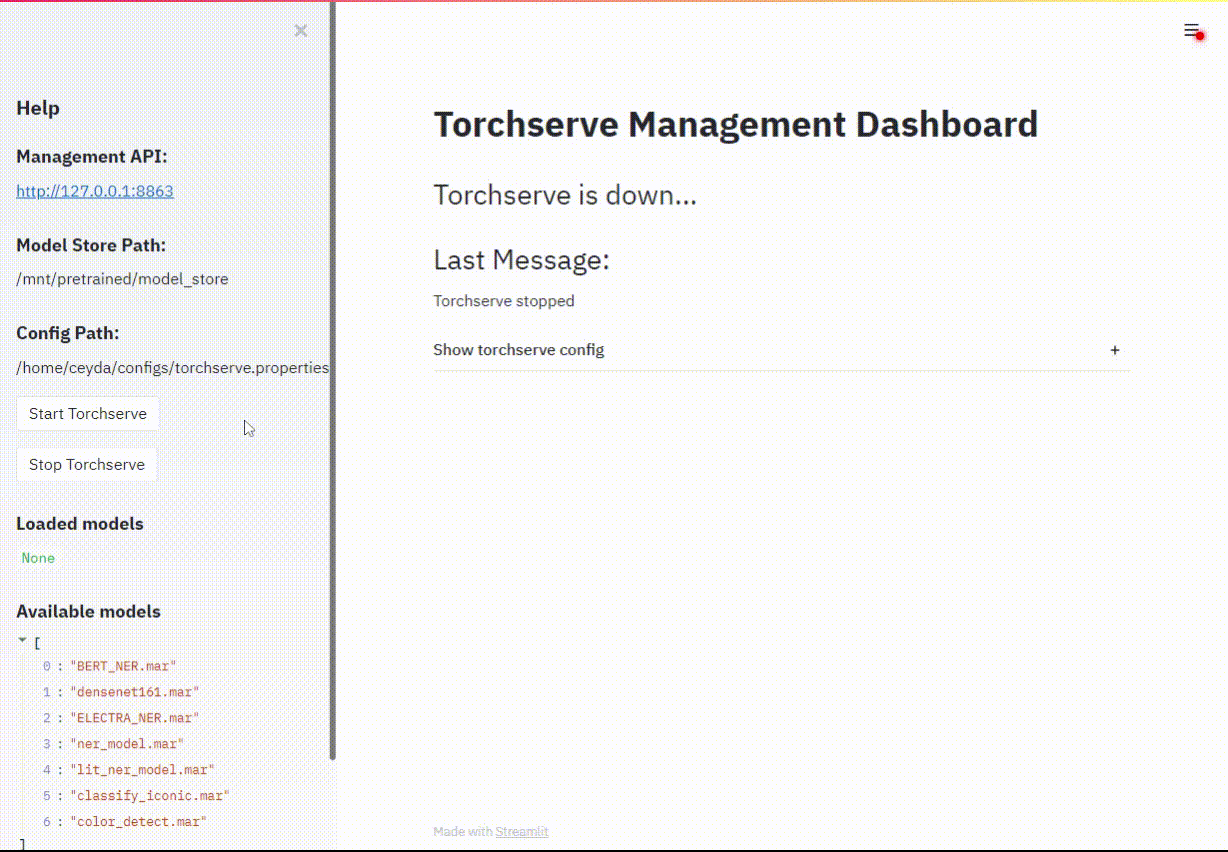
Torchserve 토치서브?
Torchserve 는 모델 서비스 배포를 도와주는 도구이다.
- 요청에 대한 자동 배치 작업? : 요청에 대한 poolers & threads가 필요하지 않다.
- 일시에 210개 요청이 서버에 왔다고 하자, 배치 크기가 16이라면 해당 요청은 (16*13)+2 형태가 된다. 즉 13개 배치는 처리되어 응답하지만 남은 2개 요청은 max_batch_delay 이전까지는 다른 요청을 기다린다. (각 request = 1 example)
- 유연한 확장성 Flexible scalability
- 다중 처리나 작업자 개수 변경이 쉽다.
- 작업이 많을 때에는 속도 향상을 위해 작업자 수를 증가시킬 수 있다.
- 어떤 이유로 작업이 멈춘 경우에 자동으로 작업자를 만든다.
- 모델 버전 관리 versioning
- 간편한 A/B 테스트
- 내장 핸들러: image_classification, object_detection, text_classification, image_segmentation 작업용 내장 핸들러
- 간단하지만 시작점으로 출발하기에 좋다.
- 측정 로그 기록 Metrics logging (상세 내용은 다른 문서 참조 바람)
- 접근 로그 Access logs, 성능 로그 performance logs, 커스텀 로그 custom logs.
사용할 모델 준비
먼저 torch-model-archiver 유틸리티를 이용하여 .mar 파일을 만들어야 한다. 이것은 압축 파일 구조에 여러분 모델을 넣는 것이다. 또한 예측 작업에 필요한 파일들도 함께 패키징되어야 한다.
torch-model-archiver 작업에 필수인 것
- 저장 모델
- 커스텀 또는 내장 핸들러
모델 저장하기
두 가지 방법이 있다.
- JIT 저장 models : torchscript 모드 이용 (아래 예제)*
- torch.save(model, PATH) 또는 torch.save(model.state_dict(), PATH)로 저장한 모델
# scripted mode
from torchvision import models
import torch
model = models.densenet161(pretrained=True)
# OR a model you saved before with torch.save(model, PATH)
# model = torch.load('/mnt/model_you_saved_before.pth')
sm = torch.jit.script(model)
sm.save("my_fancy_model.pt")
아래 방법은 더 좋다.
#traced mode
from torchvision import models
import torch
model = models.densenet161(pretrained=True)
# OR a model you saved before with torch.save(model, PATH)
# model = torch.load('/mnt/model_you_saved_before.pth')
model.eval()
example_input = torch.rand(1, 3, 224, 224)
traced_script_module = torch.jit.trace(model, example_input)
traced_script_module.save("my_fancy_model.pt")
모델 패키징 (.mar 파일)
torch-model-archiver \
--model-name my_fancy_model \ # .mar 파일명 -> my_fancy_model.mar
--version 1.0 \
--handler image_classifier \ # 기본 핸들 또는 사용자 handler.py
--serialized-file ./my_fancy_model.pt \ # 필요하지만, 핸들러 설정에도 의존한다
# context.manifest['model']['serializedFile']
--model-file ./model.py \ # 모델을 전체 torch.save(model) 또는 torchscript model 사용하면
# 필요하지 않다. (context.manifest['model']['modelFile'] 설정)
--extra-files ./index_to_name.json \ # 핸들러 동작을 위한 파일이다. 기본 핸들러는 ids를
# 분류 label에 매핑하는 index_to_name.json 파일 사용
--runtime python3 \
--export-path ./model_store \ # .mar 파일이 저장되는 장소이다.
-requirements-file \ # 다른 파이썬 패키지들을 함께 넣을 때 지정한다.
--force \ # 만약 .mar 파일이 있더라고 겹쳐쓰라는 옵션이다.
--archive-format default \ # {default,tgz,no-archive}
# -> 결과는 .mar 또는 .tar.gz 또는 폴더(비압축) 형태
기본 핸들러 Inbuilt handlers
image_classification, object_detection, text_classification, image_segmentation 작업을 위한 내장 핸들러, 즉 기본 핸들러가 제공된다. 기본 핸들러를 사용하여 간단하게 예제 모델을 관리할 수 있지만 커스터마이징을 위한 사용자 핸들러가 필요할 수 있다. 사용자 핸들러를 작성할 때는 BaseHandler를 상속하고 수정하는 것이 깔끔하다.
참고: 예제 BaseHandler:
토치서브 핸들러
초기화(한번) -> 핸들러로 제어 ( 전처리 preprocess -> 예측 inference -> 후처리 postprocess )
class BaseHandler(abc.ABC):
# 미생략 코드: https://github.com/pytorch/serve/blob/6c56b7ddee00a14fcdfab9bedf37f011e11fdece/ts/torch_handler/base_handler.py
def __init__(self):
self.model = None
def initialize(self, context):
# boilerplate
properties = context.system_properties
self.map_location = "cuda" if torch.cuda.is_available() else "cpu"
self.device = torch.device(
self.map_location + ":" + str(properties.get("gpu_id")) if torch.cuda.is_available() else self.map_location
)
self.manifest = context.manifest
# model_dir는 내부 폴더로 지정
# extra-files은 이 폴더에.
model_dir = properties.get("model_dir")
# serialized-file 옵션
# 사용자 핸들러를 작성할 때는 건너뛸 수 있음!
# (torch-model-archiver에 빈 파일을 지정할 수 있다)
serialized_file = self.manifest["model"]["serializedFile"]
model_pt_path = os.path.join(model_dir, serialized_file)
# eager model을 사용 안하는 경우 건너뛸 수 있다.
model_file = self.manifest["model"].get("modelFile", "")
if model_file:
logger.debug("Loading eager model")
self.model = self._load_pickled_model(model_dir, model_file, model_pt_path)
else:
logger.debug("Loading torchscript model")
self.model = self._load_torchscript_model(model_pt_path)
self.model.to(self.device)
self.model.eval()
def preprocess(self, data):
# data 구조: [batch_size,...]
return data
def inference(self, data, *args, **kwargs):
# data는 preprocess 반환 값이며 이것을 모델에 전달해서 예측 결과를 받는다.
result = self.model(data)
return result
def postprocess(self, data):
"""
:return: 반환 시 Python 리스트로 변경할 것! (중요함!!!)
"""
# data는 postprocess 반환 값이다.
# return 형태는 [batch_size,...]일 것이다.
return data.tolist()
def handle(self, data, context):
self.context = context
data = self.preprocess(data)
data = self.inference(data)
data = self.postprocess(data)
return data
여기에서 context는 아래 특성들을 포함한다.
context.model_name = model_name
context.manifest = manifest
context.system_properties = {
"model_dir": model_dir,
"gpu_id": gpu,
"batch_size": batch_size,
"server_name": "MMS",
"server_version": mms_version
}
context.metrics=None
Manifest: torch-model-archiver 상세 정보를 포함한다.
{'createdOn': '13/10/2020 19:34:50',
'runtime': 'python3',
'model': {
'modelName': 'my_fancy_model',
'serializedFile': 'my_fancy_model.pt',
'handler': 'serve.py',
'modelVersion': '1.0'},
'archiverVersion': '0.2.0'}
사용자 핸들러 작성하기
[참조] inbuilt handlers.
아래는 image_classifier 핸들러 일부이다. 코드에 보이듯이 단지 VisionHandler는 BaseHandler의 서브클래스이다. 이 핸들러는 여러분의 image_processing transforms(top_k 다른 설정 등)을 수행한다.
class VisionHandler(BaseHandler, ABC):
"""
Base class for all vision handlers
"""
def preprocess(self, data):
images = []
for row in data:
image = row.get("data") or row.get("body")
image = Image.open(io.BytesIO(image))
image = self.image_processing(image)
images.append(image)
return torch.stack(images)
class ImageClassifier(VisionHandler):
"""
ImageClassifier handler class. This handler takes an image
and returns the name of object in that image.
"""
topk = 5
# These are the standard Imagenet dimensions
# and statistics
image_processing = transforms.Compose([
transforms.Resize(256),
transforms.CenterCrop(224),
transforms.ToTensor(),
transforms.Normalize(mean=[0.485, 0.456, 0.406],
std=[0.229, 0.224, 0.225])
])
def set_max_result_classes(self, topk):
self.topk = topk
def get_max_result_classes(self):
return self.topk
def postprocess(self, data):
ps = F.softmax(data, dim=1)
probs, classes = torch.topk(ps, self.topk, dim=1)
probs = probs.tolist()
classes = classes.tolist()
return map_class_to_label(probs, self.mapping, classes)
서버 설정 Configuration
기본 torchserve config는 아래 config.properties 파일이다.
[config.properties]
inference_address=http://127.0.0.1:8080
management_address=http://127.0.0.1:8081
metrics_address=http://127.0.0.1:8082
number_of_gpu=0
batch_size=1
model_store=./model_store
사용할 포트 번호나 mar 파일 저장 디렉터리를 지정할 수 있다.
또한 batch_size(작업 단위 크기) 및 number_of_gpu(GPU 개수)도 원하는 적정 값으로 넣을 수 있다.
서버 실행
torchserve --start --ncs --model-store ./model_store --models my_fancy_model=my_fancy_model.mar --foreground --ts-config ./config.properties
또는 torchserve-dashboard에서 실행하기
torchserve-dashboard --server.port 8505 -- --config_path ./config.properties
웹 브라우저 접속: http://localhost:8505

사용할 .mar 파일을 선택하고, 이름을 지정한 다음에 register를 클릭한다.
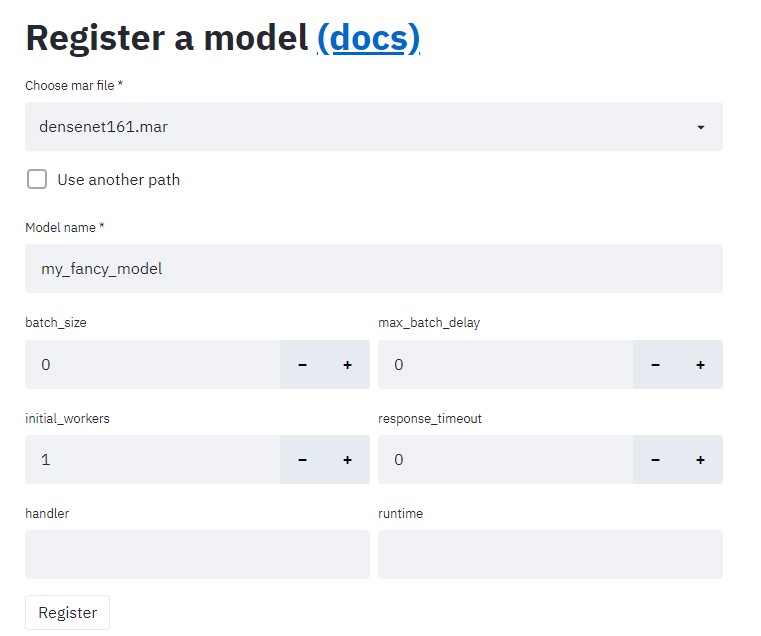
이후에 모델을 제거한다.
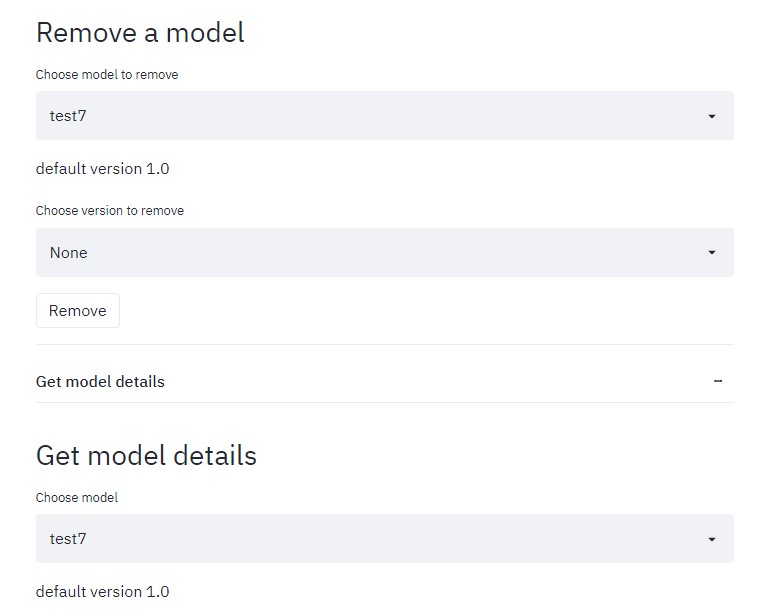
HTTP requests로 예측하기
VisionHandler에 요청 데이터를 볼 수 있다. 예측을 요청하는 파이썬 파일은 다음과 같다.
[what.py]
import io
import httpx
from PIL import Image
img_file = open("./test_img.jpg", "rb").read()
image = Image.open(io.BytesIO(img_file))
res = httpx.post("http://127.0.0.1:7863/predictions/my_fancy_model", data=img_file)
res.json()
그리고,,,
A full-blown production ready tool IMO.
- Native support for type & dimension checks. Sure, you can do all that in your custom handler but would be nice to have it built-in. For example in VisionHandler by adding a check for image dimensions.
- Plugins documentation
참조
- Torchserve는 서빙 중에 .mar 파일을 /tmp/ 디렉터리에 압축을 해제하여 사용한다.
- index_to_name.json 형식은 {"0": "cat","1": "dog"} 이다.
보안 주의: Management API/UI는 public 어드레스로 관리하지 말자(localhost 이용). https+auth 또는 ssh port forwarding을 가진 reverse proxy를 추천한다. 참조(https://pytorch.org/serve/configuration.html#configure-torchserve-listening-address-and-port)
Q&A issue.
'TorchServe' 카테고리의 다른 글
토치서브 시스템 측정 항목 (System Metrics) (0) | 2021.12.22 |
---|---|
TORCHSERVE REST API (0) | 2021.12.22 |
토치서브 명령 옵션 사용하기 (0) | 2021.12.20 |
PyTorch에서 모델 배포 (TorchServe) (0) | 2021.12.07 |
TorchServe로 PyTorch 모델 제공 방법 (영어 동영상) (0) | 2021.12.07 |